Originally published at: https://www.brainpad.com/2024/03/07/brainsafe-with-monitoring-system/
Project Overview
Deep Learning Real-Time Face Mask Detection System
Dive into the World of Face Mask Detection with the BrainPad Pulse Microcomputer and experience the fusion of advanced computer vision and hardware interaction with our innovative project on face mask detection. By harnessing the power of the BrainPad Pulse microcomputer and cutting-edge deep learning models, this project offers a real-time alert system to promote safety measures in public spaces.

How It Works
Our project utilizes state-of-the-art deep learning models for face detection and mask-wearing classification, allowing it to identify individuals wearing masks accurately. The BrainPad Pulse microcomputer serves as the interface for providing visual and auditory alerts when someone is detected without a mask, promoting adherence to safety protocols.
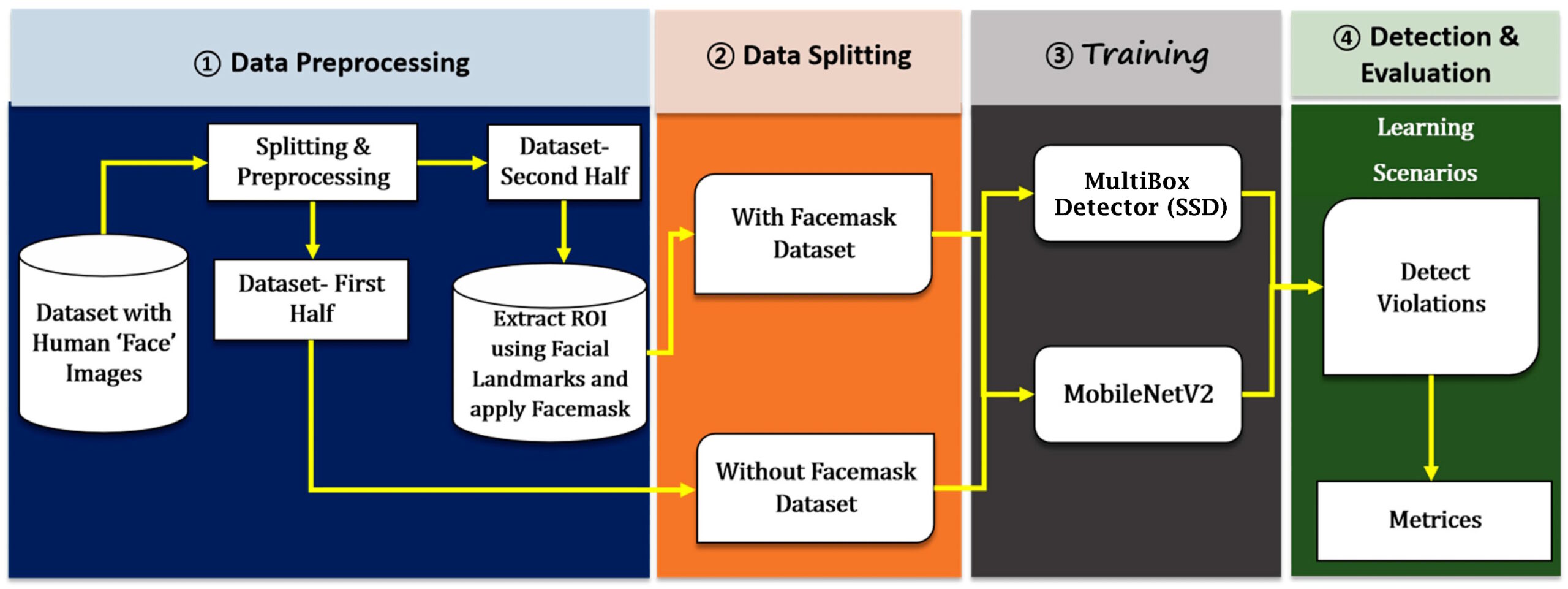
Project Features
Here are the key features that make our project indispensable:
- Face Detection: Utilizing a pre-trained deep learning model based on the Single Shot MultiBox Detector (SSD) framework with MobileNetV2 architecture, our system accurately detects faces in real-time video streams, ensuring reliable mask detection.
- Mask Detection: With another pre-trained deep learning model, our system classifies whether each detected face is wearing a mask or not. This model has been trained on a diverse dataset of images featuring individuals with and without masks, ensuring robust performance across different scenarios.
- Real-Time Video Processing: Our system continuously captures frames from a video stream, processes them using the face and mask detection models, and displays the results in real time. This seamless integration allows for quick and efficient monitoring of public spaces.
- Feedback via Hardware Interaction: Enhancing user engagement and awareness, our system integrates with the BrainPad Pulse microcomputer to provide real-time feedback when individuals are detected without wearing masks. The BrainPad Pulse’s LED and LCD are utilized to deliver visual and auditory alerts, prompting individuals to adhere to mask-wearing guidelines.
- Customizable Threshold: To accommodate varying confidence levels and environmental conditions, our system allows users to customize confidence thresholds for face and mask detection. This flexibility ensures adaptability to different settings and enhances the system’s versatility.
Hardware Requirements
To launch this exciting project, you’ll need the following hardware components:
- BrainPad Pulse Microcomputer: The central component responsible for processing data and controlling the alert system.
- Webcam or Video Stream Source: Provides live input for face detection and mask classification.
Software Requirements and Instructions
Get started on your face mask detection journey by following these steps:
- Clone Repository: Start cloning the FaceMaskDetection repository from our GitHub to access the project code and resources.
- Install Required Python Libraries: Ensure you have the necessary Python libraries installed by executing in your terminal.
pip install numpy opencv-python imutils tensorflow keras DUELink
- Connect Hardware: Connect your webcam or video stream source to your computer, ensuring it’s functioning correctly.
- Run the Script: Open a terminal window or your IDE, navigate to the project directory, and run the
detect_mask_video.py
script using Python. - Experience Real-time Alert System: Witness the real-time detection of faces and classification of mask usage. When someone is detected without a mask, the BrainPad Pulse microcomputer will provide visual and auditory alerts to remind them to wear one, promoting safety in public spaces.
Code Overview
Let us break down the Python code into smaller steps and provide a comprehensive explanation for each part:
# Import required libraries
from keras.applications.mobilenet_v2 import preprocess_input
from keras.preprocessing.image import img_to_array
from keras.models import load_model
import imutils
from imutils.video import VideoStream
import numpy as np
import time
import cv2
# Import custom library for BrainPad interaction
from DUELink.DUELinkController import DUELinkController
# Obtain available port for connection and initialize BrainPad controller
available_port = DUELinkController.GetConnectionPort()
brain_pad_controller = DUELinkController(available_port)
# Load pre-trained face detector model
print("[INFO] Loading face detector model...")
prototxt_path = "face_detector/deploy.prototxt"
weights_path = "face_detector/res10_300x300_ssd_iter_140000.caffemodel"
face_net = cv2.dnn.readNet(prototxt_path, weights_path)
# Load pre-trained face mask detector model
print("[INFO] Loading face mask detector model...")
mask_net = load_model("mask_detector.model")
# Set minimum confidence threshold to filter weak detections
confidence_threshold = 0.5
# Initialize video stream and allow camera sensor to warm up
print("[INFO] Starting video stream...")
video_stream = VideoStream(src=0).start()
time.sleep(2.0)
# Clear BrainPad display and show initial state
brain_pad_controller.Display.Clear(0)
brain_pad_controller.Display.Show()
def detect_and_predict_mask(frame, face_net, mask_net):
# Obtain frame dimensions and construct blob
(h, w) = frame.shape[:2]
blob = cv2.dnn.blobFromImage(frame, 1.0, (300, 300), (104.0, 177.0, 123.0))
# Pass blob through face detection network and obtain detections
face_net.setInput(blob)
detections = face_net.forward()
# Initialize lists for faces, their locations, and mask predictions
faces = []
locs = []
preds = []
# Loop over detections
for i in range(0, detections.shape[2]):
# Extract confidence associated with detection
confidence = detections[0, 0, i, 2]
# Filter out weak detections
if confidence > confidence_threshold:
# Compute bounding box coordinates
box = detections[0, 0, i, 3:7] * np.array([w, h, w, h])
(start_x, start_y, end_x, end_y) = box.astype("int")
# Ensure bounding boxes fall within frame dimensions
(start_x, start_y) = (max(0, start_x), max(0, start_y))
(end_x, end_y) = (min(w - 1, end_x), min(h - 1, end_y))
# Extract face region, preprocess it, and add to lists
face = frame[start_y:end_y, start_x:end_x]
if face.any():
face = cv2.cvtColor(face, cv2.COLOR_BGR2RGB)
face = cv2.resize(face, (224, 224))
face = img_to_array(face)
face = preprocess_input(face)
faces.append(face)
locs.append((start_x, start_y, end_x, end_y))
# Make predictions if at least one face was detected
if len(faces) > 0:
faces = np.array(faces, dtype="float32")
preds = mask_net.predict(faces, batch_size=32)
return locs, preds
# Main loop for processing video stream frames
while True:
# Capture frame from video stream and resize
frame = video_stream.read()
frame = imutils.resize(frame, width=800)
# Detect faces and predict if they are wearing masks
(locs, preds) = detect_and_predict_mask(frame, face_net, mask_net)
# Loop over detected faces and their predictions
for (box, pred) in zip(locs, preds):
# Unpack bounding box and predictions
(start_x, start_y, end_x, end_y) = box
(mask, without_mask) = pred
# Determine class label and color for bounding box
label = "Mask" if mask > without_mask else "No Mask"
color = (0, 255, 0) if label == "Mask" else (0, 0, 255)
# Toggle BrainPad Pulse LED and display message for "No Mask" detection
if label == "No Mask":
brain_pad_controller.Frequency.Write('p', 1000, 100, 100)
brain_pad_controller.Display.DrawTextScale("Wear a Mask", 1, 0, 10, 2, 2)
brain_pad_controller.Display.Show()
brain_pad_controller.Led.Set(200, 200, -1)
brain_pad_controller.Display.Clear(0)
brain_pad_controller.Display.Show()
brain_pad_controller.Led.Set(0, 0, -1)
# Include probability in label
label = f"{label}: {max(mask, without_mask) * 100:.2f}%"
# Display label and bounding box on frame
cv2.putText(frame, label, (start_x, start_y - 10),
cv2.FONT_HERSHEY_SIMPLEX, 0.45, color, 2)
cv2.rectangle(frame, (start_x, start_y), (end_x, end_y), color, 2)
# Show output frame
cv2.imshow("Frame", frame)
key = cv2.waitKey(1) & 0xFF
# Break from loop if 'q' key pressed
if key == ord("q"):
break
# Cleanup
cv2.destroyAllWindows()
video_stream.stop()
Customization:
- Alert System Enhancements:
Explore ways to enhance the alert system by customizing the visual and auditory feedback provided by the BrainPad Pulse microcomputer. You can modify the alert messages on LCD, onboard LED, and sound effects to suit different environments and preferences.
- Project Optimization:
Experiment with optimization techniques to improve the performance and efficiency of the detection process by adding some modules and sensors to the projects from BrainClip or BrainTronics kit like motion, light, or distance sensors and servo to control doors or more LEDs as a Nepixel.
- Explore Different microcomputers with BrainPad :
Try rewriting the project code in alternative microcomputers using the BrainPad Edge or BrainPad Rave. and also, Explore how the project behaves with different microcomputers.
Image courtesy of Peyman Majidi Mask On Face Detection